Vn-Z.vn On November 16, 2023, the scientific journal Science published an article stating that Google has upgraded its DeepMind weather forecasting model to the most powerful prediction ability ever. In just under 1 minute, this model can directly predict the weather for the next 10 days.
Evaluated with an accuracy level of up to 90%, this AI model surpasses the most advanced human systems, marking the most significant advancement in AI weather forecasting!
Soucre: Science
Previously, DeepMind accurately predicted rainfall amounts within 1-2 hours. Short-term weather forecasting is crucial for managing water resources, agriculture, aviation, emergency planning, outdoor activities, and supporting various sectors that heavily rely on weather for decision-making.
Recently, the DeepMind weather forecasting development team continued their research on a new forecasting technology called GraphCast, which has been published on the open-source platform Github.
This technology uses a resolution of 0.25 degrees longitude/latitude (equivalent to about 28x28 km at the equator), surpassing the current highest resolution of 1 degree. This resolution divides the Earth's surface into over a million grids, each capable of generating hundreds of forecast data points, totaling tens of millions of data points.
Unlike traditional forecasting methods, GraphCast primarily relies on data patterns for predictions rather than using human-generated physical equations. Compared to the human-created HRES forecasting technology, GraphCast outperforms it by 90% out of 1380 test indices. When limited to atmospheric layers, GraphCast surpasses HRES in 99.7% of indices.
Across more than 1 million grids, each GraphCast grid can generate 227 prediction data. It includes 6 atmospheric variables (including specific humidity, wind speed and direction, and temperature) at each altitude at 37 different altitudes. On Earth's surface, GraphCast can also predict five variables including temperature, wind speed and direction, and average sea level pressure.
The full variation types and specific altitudes (expressed in air pressure, unit: hPa) are shown in the following table:
The number in parentheses in the column headings is the number of entries in that column. Bold variables and levels indicate which variables and levels were included in the scorecard evaluation. All atmospheric variables are represented at each pressure level.
To compare the performance of GraphCast and HRES, researchers selected historical data from 2018 (GraphCast's training data ended in 2017) from ERA5 reanalysis data by the European Centre for Medium-Range Weather Forecasts (ECMWF).
Researchers requested HRES and GraphCast to provide "predictions" in the current situation and then compared their "predictions" with ERA5.
At the 500hPa level, the RMSE (Root Mean Square Error, lower values indicate better performance) and ACC (Anomaly Correlation Coefficient) scores of GraphCast were significantly better than HRES.
Among the 1380 data points at 50-1000hPa selected by researchers, GraphCast outperformed HRES in 90.3% of cases, with a significant advantage in 89.9% (in the group 'd' in the figure below, where green indicates GraphCast outperforming HRES and darker shades indicate a clearer advantage).
Image: Science.org
Beyond these data, GraphCast exhibits distinct advantages in predicting severe weather.
For the trajectory of a tropical storm, GraphCast shows lower average errors than HRES, notably starting from the initial 4.75 days when the advantage becomes evident (a,b below).
In predicting the atmospheric river's moisture stream, GraphCast's RMSE values are significantly lower than HRES (Figure c below).
In forecasting heatwaves, GraphCast is also more accurate than HRES at 12 hours, 5 days, and 10 days ahead (Figure d below).
A) Tornado tracking performance for GraphCast and HRES. The x-axis represents the execution time (in days) and the y-axis represents the average path error (in km). Error bars represent bootstrapped 95% confidence intervals for the mean. (B) Difference in tornado track coupling error between GraphCast and HRES. The x-axis represents the execution time (in days) and the y-axis represents the average pairing error difference (in km). Error bars represent bootstrapped 95% confidence intervals for the mean difference (see supplementary material 8.1). (C) Atmospheric river prediction (IVT) skill for GraphCast and HRES. The x-axis represents execution time (in days) and the y-axis represents RMSE. Error bars are 95% confidence intervals. (D) Accurate recall of extreme temperature predictions for GraphCast and HRES. The x-axis represents recall and the y-axis represents precision. The curves represent the different precision-recall trade-offs when scanning over the gain applied to the forecast signal (see supplementary material section 8.3). (Source Science.org)
In September 2023, GraphCast accurately predicted Hurricane Lee in the North Atlantic nine days before the storm made landfall, compared to six days before using traditional methods.
Zoom Earth
Storm Lee was confirmed to be one of the rare storms that increased in intensity incredibly quickly, in just 24 hours it increased from Level 1 to Level 5 (according to the US scale). Not only that, it also has continuous changes in intensity, very strange and unpredictable.
GraphCast not only has high accuracy but also has the ability to predict very quickly. A 10-day forecast using GraphCast on a Google TPU v4 machine takes less than a minute to complete.
While using traditional methods like HRES takes several hours even on supercomputers.
GraphCast achieves effective and accurate weather forecasts without using physical equations, this technology relies entirely on data analysis.
In the workflow, enter weather data from 6 hours ago to the present time and GraphCast can predict the weather for the next 6 hours.
Forecast data can be used as a new "current" state to continue iterative predictions and can predict weather conditions up to 10 days later.
For input data, GraphCast only requires two datasets: the weather state 6 hours ago and the current weather state. The model then predicts the weather 6 hours into the future. This can then be deployed in 6-hour intervals to provide state-of-the-art forecasts up to 10 days in advance. (Image deepmind.google
Basically, GraphCast uses machine learning and graph neural network (GNN) architecture, including an encoding layer and a decoding layer, along with 16 intermediate layers, with a total of 36.7 million parameters. number. It only uses existing meteorological data to make predictions, not relying on human-built physical equations.
GraphCast maps meteorological data on a 0.25 degree grid into a neural network, then the calculation results are passed and reconstructed back into meteorological data through the decoding layer.
Trong quá trình huấn luyện, GraphCast sử dụng dữ liệu tái phân tích thời tiết gần 40 năm từ tập dữ liệu ERA5 (1979-2017), bao gồm hình ảnh vệ tinh, radar và kết quả đo lường từ các trạm khí tượng.
ERA5 được tạo ra từ phương pháp 4DVar và đồng hóa quan sát, tạo ra dữ liệu tái tạo tối ưu toàn cầu, bao gồm thời gian từ những năm 1940 đến hiện tại, với phạm vi toàn cầu. Nếu sử dụng dữ liệu mới gần đây hơn, kết quả dự đoán của GraphCast có thể tiếp tục cải thiện độ chính xác.
Mỗi đường màu biểu thị GraphCast được huấn luyện với dữ liệu kết thúc trước một năm khác nhau, từ năm 2018 (màu xanh) đến năm 2021 (màu tím). Trục y biểu thị điểm kỹ năng RMSE trên dữ liệu kiểm tra năm 2021, cho Z500, so với GraphCast được huấn luyện trước năm 2018, qua các khoảng thời gian dẫn (trục x). Đường đứt đứt đứt biểu thị 3.5 ngày, nơi dự báo HRES 06z/18z kết thúc. Đường đen biểu thị HRES, với các khoảng thời gian trước và sau 3.5 ngày lần lượt từ khởi đầu 06z/18z và 00z/12z. ( Ảnh Science.org)
Google DeepMind as a complement to existing weather forecasting techniques. "Our method should not be considered a replacement for traditional weather forecasting methods, which have been developed over decades and are rigorously tested," the development team said. in many real-world contexts and offers many features that we have yet to explore."
In the future, ECMWF plans to develop its own artificial intelligence model and explore integrating it into its digital weather forecasting system. The UK Met Office, in partnership with the Alan Turing Institute, is also developing graph neural networks for weather forecasting to be integrated into their supercomputing infrastructure in the future.
Vn-Z.vn team compiled and referenced sources Science.org, Google Deepmind
Evaluated with an accuracy level of up to 90%, this AI model surpasses the most advanced human systems, marking the most significant advancement in AI weather forecasting!
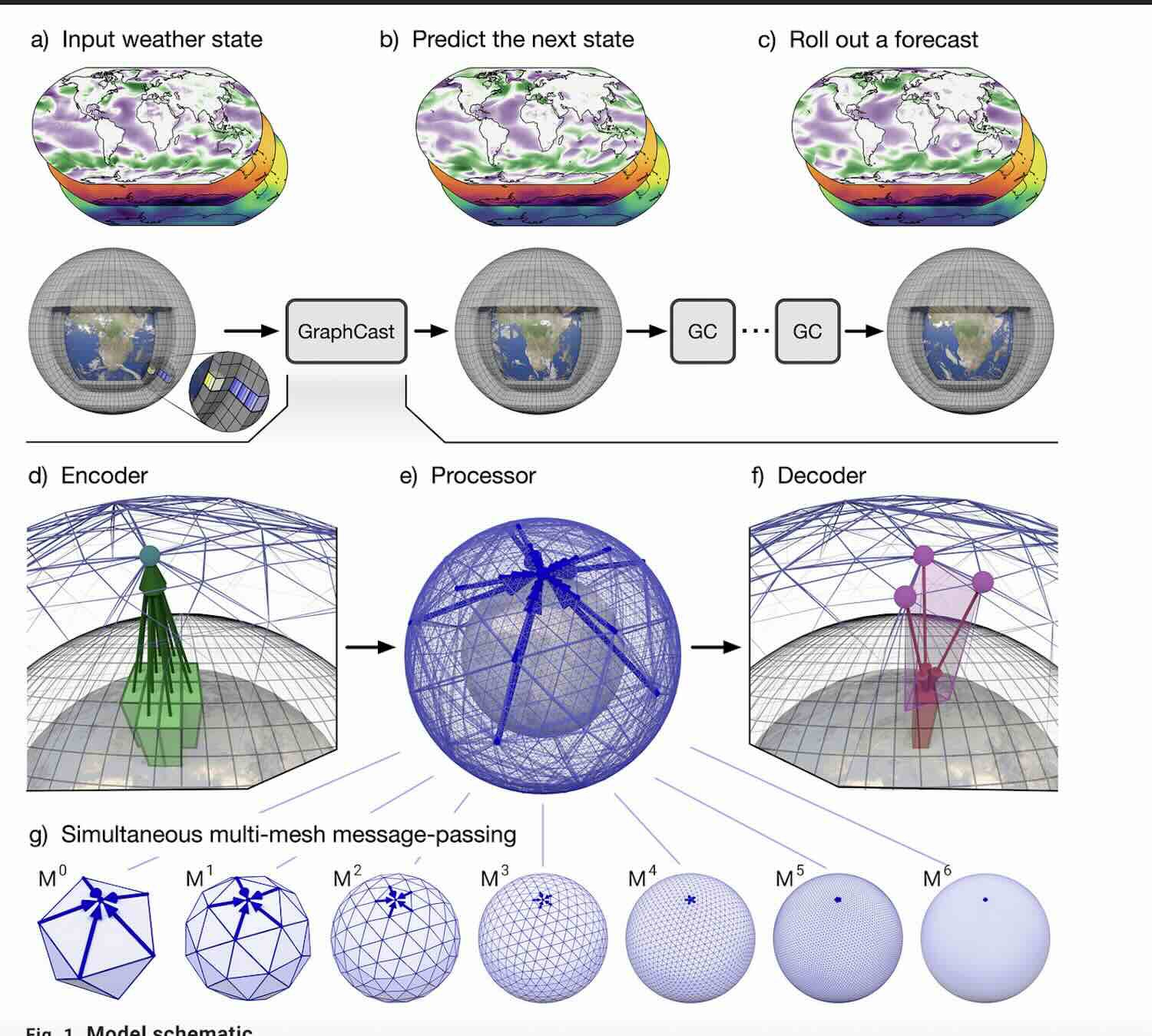
Soucre: Science
Recently, the DeepMind weather forecasting development team continued their research on a new forecasting technology called GraphCast, which has been published on the open-source platform Github.
This technology uses a resolution of 0.25 degrees longitude/latitude (equivalent to about 28x28 km at the equator), surpassing the current highest resolution of 1 degree. This resolution divides the Earth's surface into over a million grids, each capable of generating hundreds of forecast data points, totaling tens of millions of data points.
Unlike traditional forecasting methods, GraphCast primarily relies on data patterns for predictions rather than using human-generated physical equations. Compared to the human-created HRES forecasting technology, GraphCast outperforms it by 90% out of 1380 test indices. When limited to atmospheric layers, GraphCast surpasses HRES in 99.7% of indices.
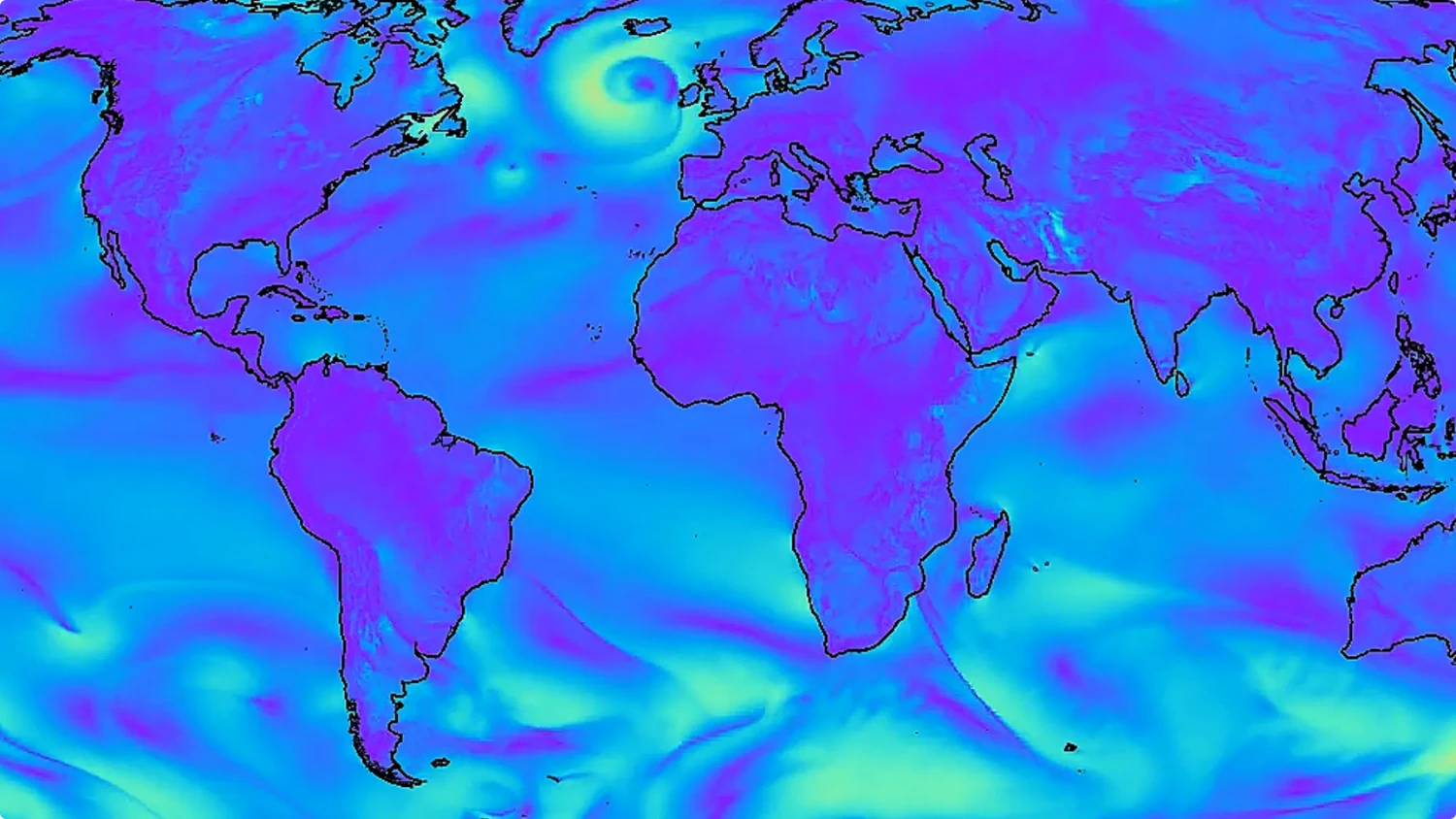
Across more than 1 million grids, each GraphCast grid can generate 227 prediction data. It includes 6 atmospheric variables (including specific humidity, wind speed and direction, and temperature) at each altitude at 37 different altitudes. On Earth's surface, GraphCast can also predict five variables including temperature, wind speed and direction, and average sea level pressure.
The full variation types and specific altitudes (expressed in air pressure, unit: hPa) are shown in the following table:

The number in parentheses in the column headings is the number of entries in that column. Bold variables and levels indicate which variables and levels were included in the scorecard evaluation. All atmospheric variables are represented at each pressure level.
Researchers requested HRES and GraphCast to provide "predictions" in the current situation and then compared their "predictions" with ERA5.
At the 500hPa level, the RMSE (Root Mean Square Error, lower values indicate better performance) and ACC (Anomaly Correlation Coefficient) scores of GraphCast were significantly better than HRES.
Among the 1380 data points at 50-1000hPa selected by researchers, GraphCast outperformed HRES in 90.3% of cases, with a significant advantage in 89.9% (in the group 'd' in the figure below, where green indicates GraphCast outperforming HRES and darker shades indicate a clearer advantage).
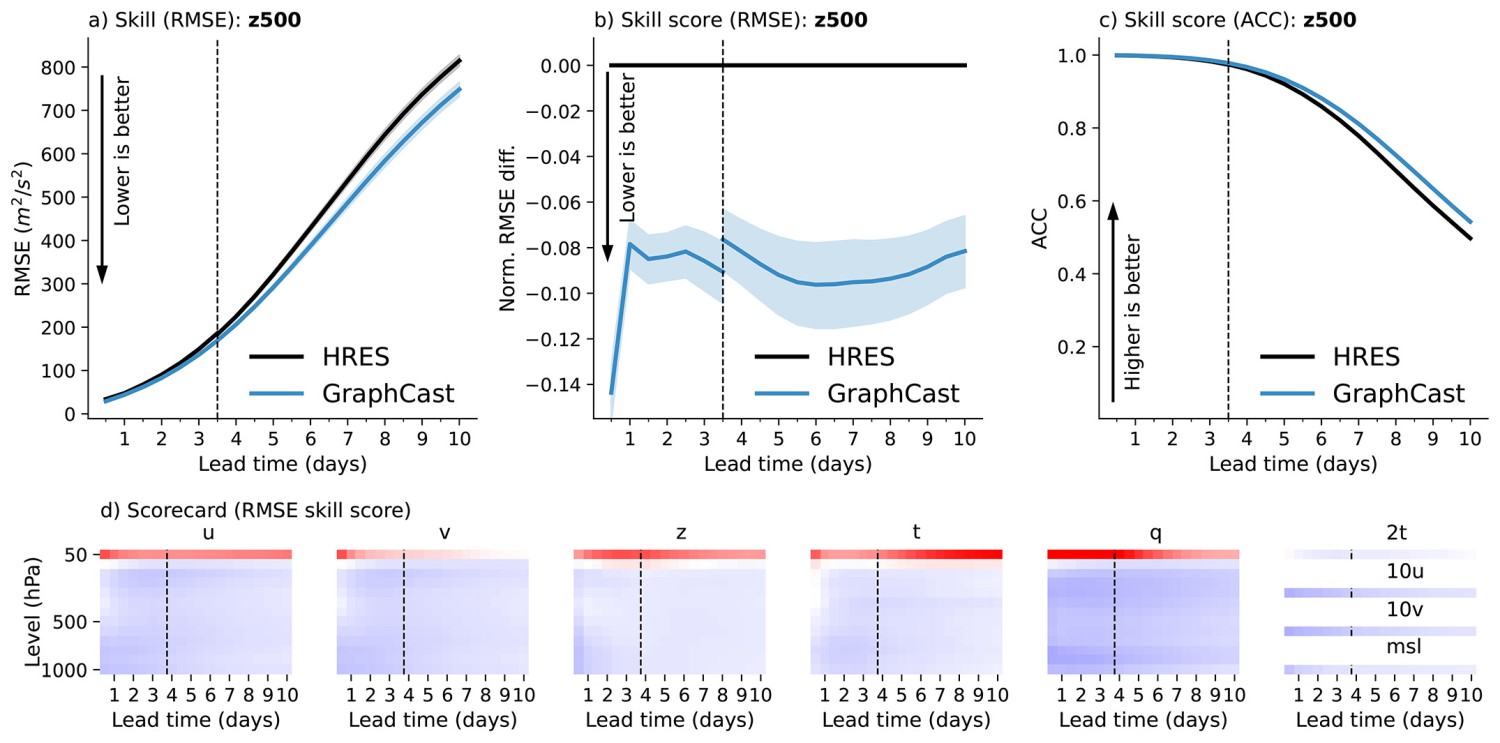
Image: Science.org
Beyond these data, GraphCast exhibits distinct advantages in predicting severe weather.
For the trajectory of a tropical storm, GraphCast shows lower average errors than HRES, notably starting from the initial 4.75 days when the advantage becomes evident (a,b below).
In predicting the atmospheric river's moisture stream, GraphCast's RMSE values are significantly lower than HRES (Figure c below).
In forecasting heatwaves, GraphCast is also more accurate than HRES at 12 hours, 5 days, and 10 days ahead (Figure d below).
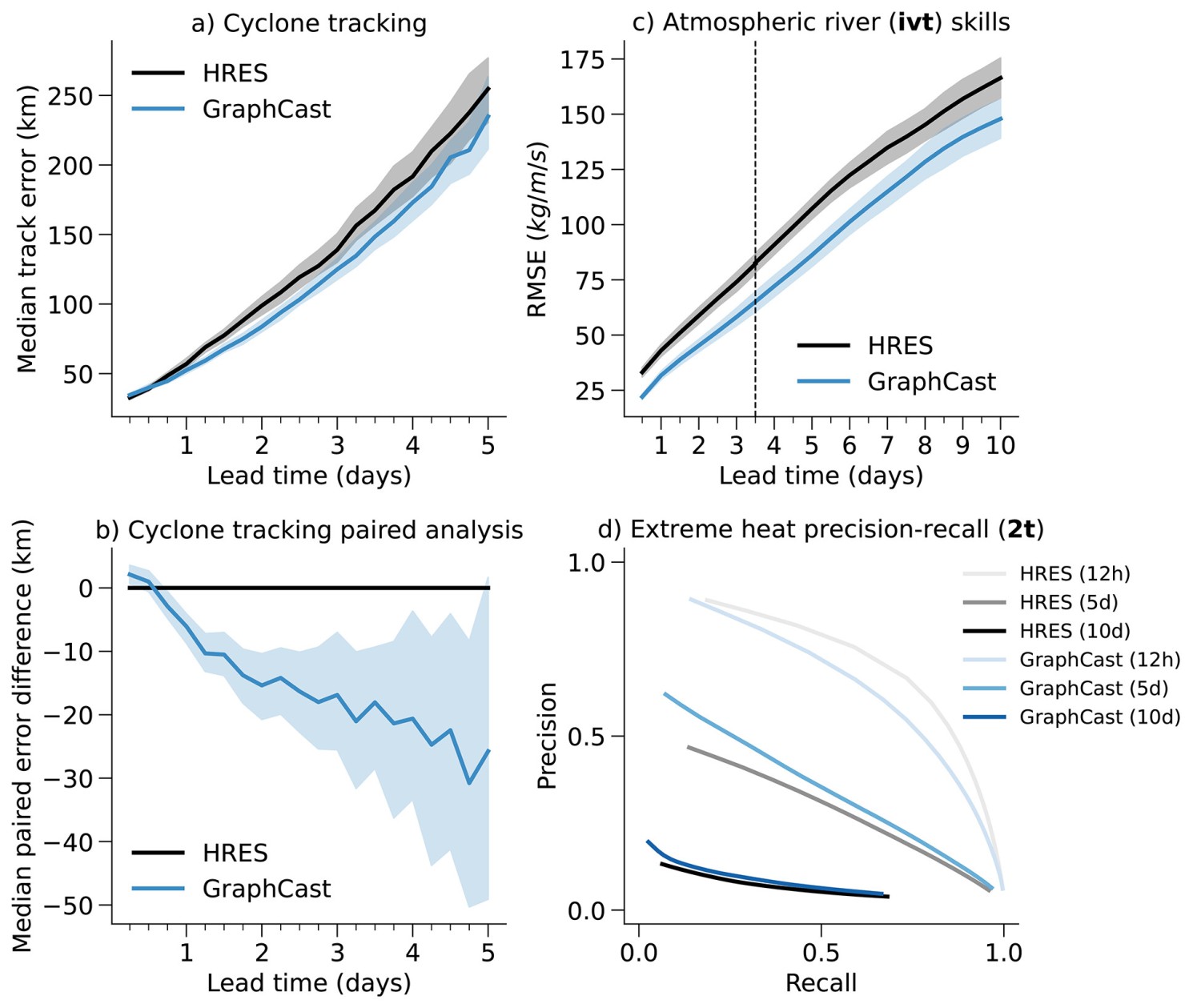
A) Tornado tracking performance for GraphCast and HRES. The x-axis represents the execution time (in days) and the y-axis represents the average path error (in km). Error bars represent bootstrapped 95% confidence intervals for the mean. (B) Difference in tornado track coupling error between GraphCast and HRES. The x-axis represents the execution time (in days) and the y-axis represents the average pairing error difference (in km). Error bars represent bootstrapped 95% confidence intervals for the mean difference (see supplementary material 8.1). (C) Atmospheric river prediction (IVT) skill for GraphCast and HRES. The x-axis represents execution time (in days) and the y-axis represents RMSE. Error bars are 95% confidence intervals. (D) Accurate recall of extreme temperature predictions for GraphCast and HRES. The x-axis represents recall and the y-axis represents precision. The curves represent the different precision-recall trade-offs when scanning over the gain applied to the forecast signal (see supplementary material section 8.3). (Source Science.org)
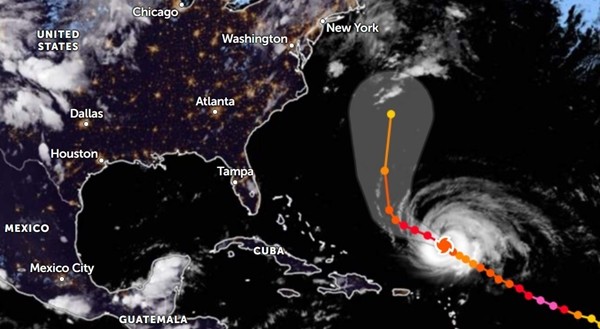
Zoom Earth
GraphCast not only has high accuracy but also has the ability to predict very quickly. A 10-day forecast using GraphCast on a Google TPU v4 machine takes less than a minute to complete.
While using traditional methods like HRES takes several hours even on supercomputers.
GraphCast achieves effective and accurate weather forecasts without using physical equations, this technology relies entirely on data analysis.
In the workflow, enter weather data from 6 hours ago to the present time and GraphCast can predict the weather for the next 6 hours.
Forecast data can be used as a new "current" state to continue iterative predictions and can predict weather conditions up to 10 days later.
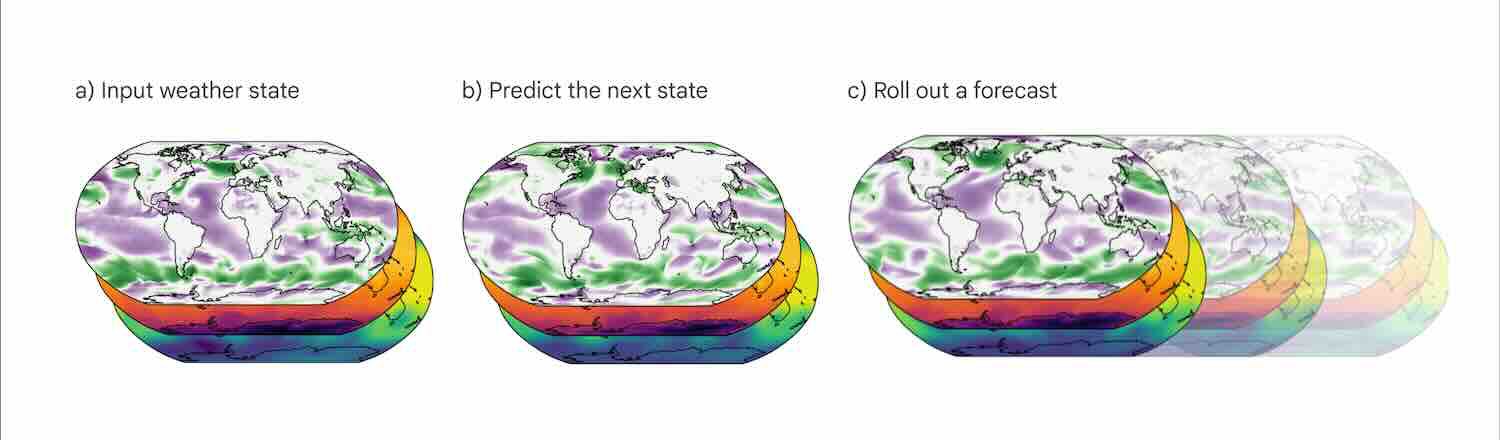
For input data, GraphCast only requires two datasets: the weather state 6 hours ago and the current weather state. The model then predicts the weather 6 hours into the future. This can then be deployed in 6-hour intervals to provide state-of-the-art forecasts up to 10 days in advance. (Image deepmind.google
GraphCast maps meteorological data on a 0.25 degree grid into a neural network, then the calculation results are passed and reconstructed back into meteorological data through the decoding layer.
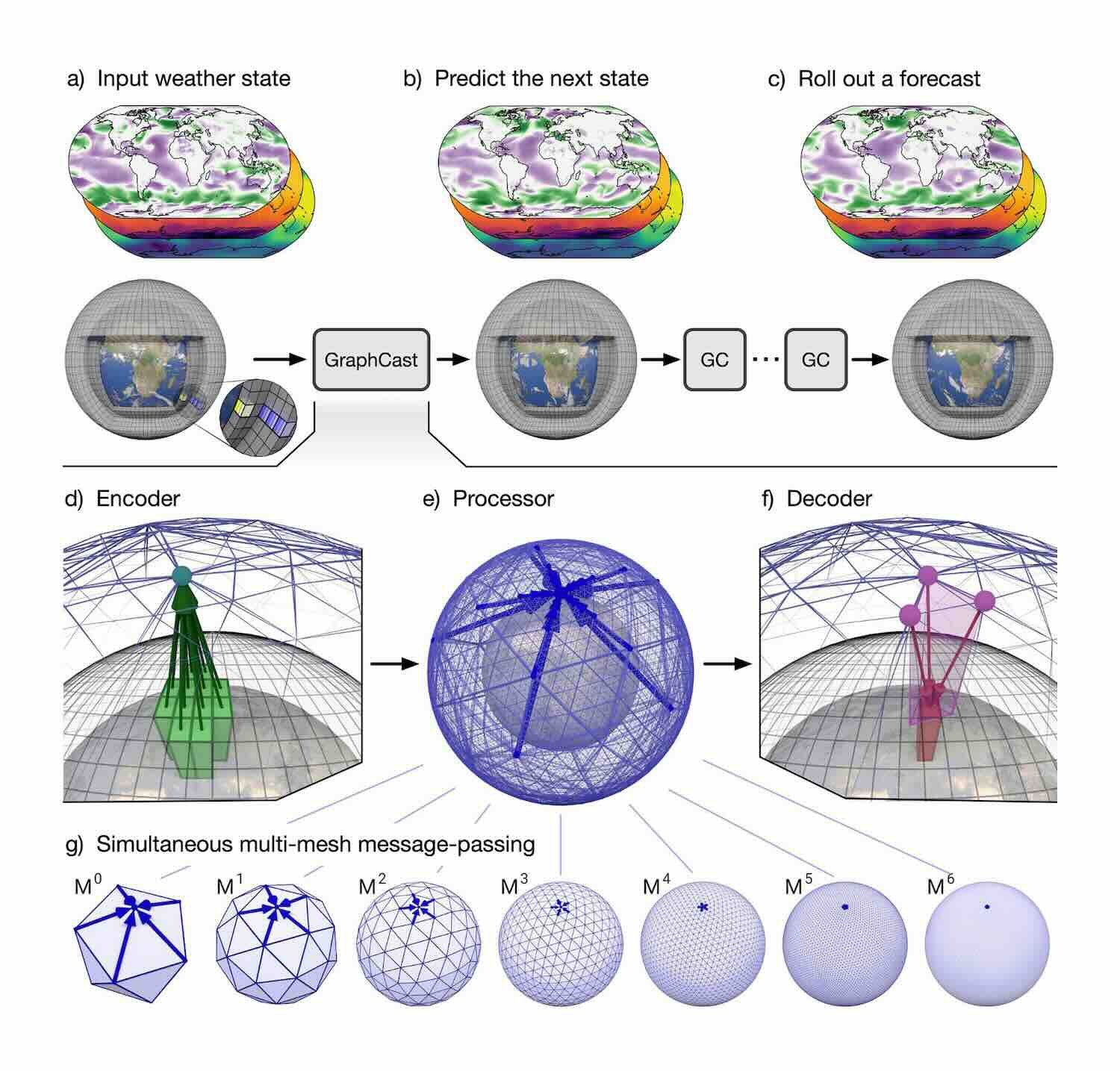
ERA5 được tạo ra từ phương pháp 4DVar và đồng hóa quan sát, tạo ra dữ liệu tái tạo tối ưu toàn cầu, bao gồm thời gian từ những năm 1940 đến hiện tại, với phạm vi toàn cầu. Nếu sử dụng dữ liệu mới gần đây hơn, kết quả dự đoán của GraphCast có thể tiếp tục cải thiện độ chính xác.
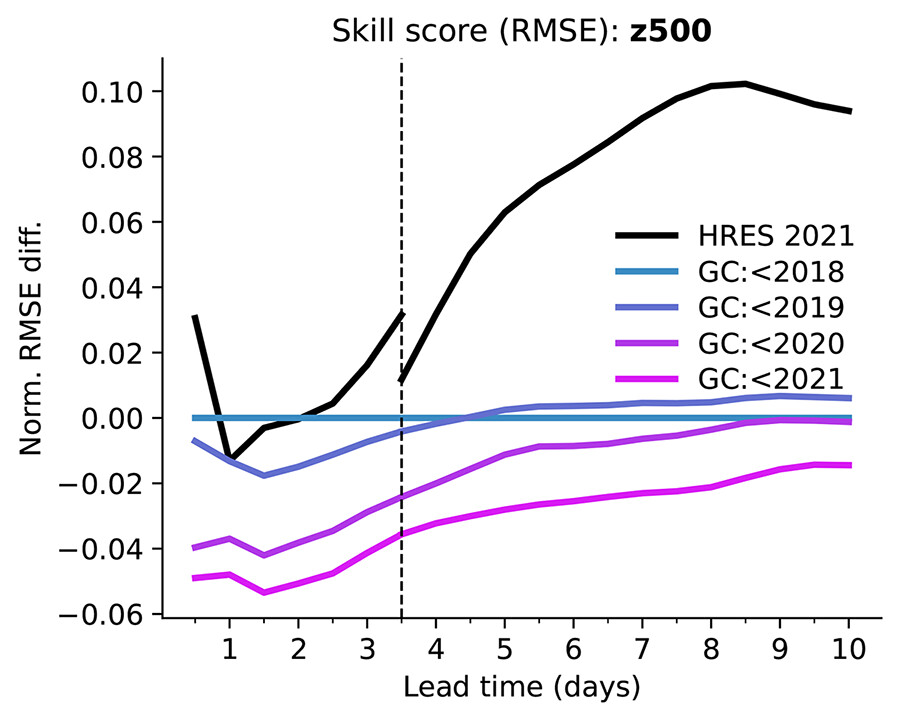
Mỗi đường màu biểu thị GraphCast được huấn luyện với dữ liệu kết thúc trước một năm khác nhau, từ năm 2018 (màu xanh) đến năm 2021 (màu tím). Trục y biểu thị điểm kỹ năng RMSE trên dữ liệu kiểm tra năm 2021, cho Z500, so với GraphCast được huấn luyện trước năm 2018, qua các khoảng thời gian dẫn (trục x). Đường đứt đứt đứt biểu thị 3.5 ngày, nơi dự báo HRES 06z/18z kết thúc. Đường đen biểu thị HRES, với các khoảng thời gian trước và sau 3.5 ngày lần lượt từ khởi đầu 06z/18z và 00z/12z. ( Ảnh Science.org)
In the future, ECMWF plans to develop its own artificial intelligence model and explore integrating it into its digital weather forecasting system. The UK Met Office, in partnership with the Alan Turing Institute, is also developing graph neural networks for weather forecasting to be integrated into their supercomputing infrastructure in the future.
Vn-Z.vn team compiled and referenced sources Science.org, Google Deepmind